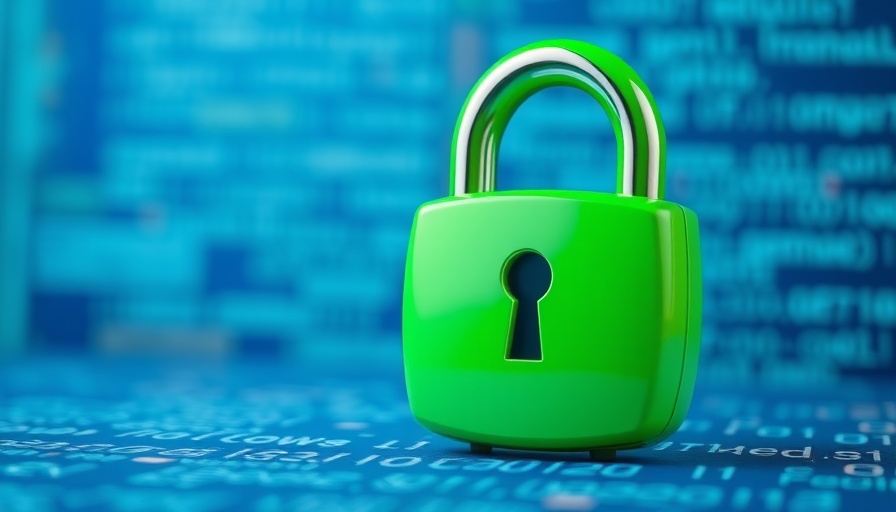
Critical Security Risks in Public Datasets for AI Training
A recent discovery has raised significant alarms within the tech community: over 12,000 live API keys and passwords were found in datasets exploited for training large language models (LLMs). Conducted by Truffle Security, the analysis of a December 2024 archive from Common Crawl unveiled these vulnerabilities hidden in what seems like a benign repository of web data that spans 18 years. The dataset, comprising 400 terabytes of information, emphasizes how unguarded credentials can compromise security measures, allowing malicious actors access to sensitive systems.
Researchers pointed out that the 219 types of secrets uncovered, ranging from Amazon Web Services (AWS) root keys to Mailchimp API keys, pose a heightened risk. Security researcher Joe Leon warned that LLMs cannot differentiate between valid and invalid secrets during their training processes, which could inadvertently foster insecure coding practices among developers. "Live" secrets here refer to any credentials that enable successful authentication, underscoring the precarious landscape in which developers operate.
Data Leakage: Subsequent Risks and Threats
The implications extend beyond just credential exposure. A warning from Lasso Security highlighted a troubling phenomenon known as Wayback Copilot, where data from public repositories could remain accessible through AI chatbots like Microsoft Copilot, even post-privacy adjustments. This situation drastically escalates the risk to organizations, with 20,580 repositories tied to notable companies such as Google, Microsoft, and IBM found to expose malicious content, including API tokens and keys.
Data poisoning attacks have also emerged as a direct threat, where attackers stealthily manipulate the integrity of datasets used for training AI systems. By tampering with the data, they skew the results and degrade LLM performance, compromising the value they provide. This trend demonstrates a critical intersection of cyber threats and AI, underscoring the necessity for robust data governance frameworks.
Proactive Measures: Securing AI Models
In light of these revelations, organizations must reassess their cybersecurity protocols concerning API handling and dataset management. Key preventive strategies include implementing stringent access controls and ensuring thorough auditing of the datasets utilized for training models. Ongoing vigilance in monitoring potential vulnerabilities will be imperative to safeguard sensitive information.
In conclusion, as LLM technology continues to evolve, so too must our approach to cybersecurity. Awareness of these vulnerabilities and implementing best practices can greatly mitigate risk and strengthen the security stance against emerging threats. Hence, businesses and developers should prioritize educating themselves about API security and remain vigilant as AI's impact broadens.
Write A Comment