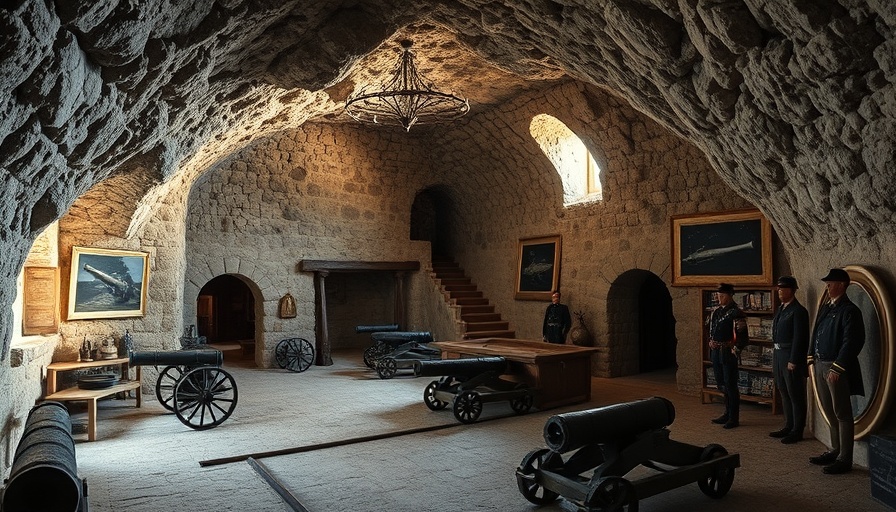
Revolutionizing AI Training with Intel’s Tiber™ Secure Federated AI
Intel's launch of the Tiber Secure Federated AI service marks a significant advancement in the AI landscape, particularly for industries such as healthcare and finance that deal with sensitive data. Unlike traditional methods where data is centralized for AI training, Tiber enables AI models to securely access data sources through a secure tunnel while the data remains in its original location. This innovative approach not only enhances data privacy but also facilitates collaboration among organizations without compromising sensitive information.
The Importance of Data Privacy in AI Training
In a world where data breaches are commonplace, the need for robust privacy measures has never been more pressing. Intel’s Tiber service taps into this necessity by using hardware and software mechanisms to establish a secure environment for AI training. According to Rajan Panchanathan, head of product at Intel's Trust and Security Services, this service allows companies to deploy advanced AI technologies while ensuring data privacy and trustworthiness in multi-party collaborations.
Real-World Applications for Diverse Fields
The Tiber service shines in sectors like banking and healthcare. By enabling banks to collaboratively enhance their fraud detection systems, Tiber allows each institution to train AI models on their local data while improving a shared AI model. Similarly, in healthcare, collaborative research can be conducted without exposing sensitive patient information. Such use cases illustrate the potential for federated AI to enhance outcomes across diverse fields while respecting individual data environments.
Challenges and Best Practices for Collaborative AI Training
While the advantages of Tiber are clear, there exist significant challenges. Effective collaboration depends heavily on the quality and uniformity of data from all participants. Inconsistent data can skew AI model accuracy, necessitating standardization efforts. Furthermore, organizations must maintain clear lines of communication regarding shared goals, timelines, and protocols. Without these, the potential of Tiber’s collaborative framework remains underutilized.
Conclusion: Embracing the Future of AI Security
Intel's Tiber Secure Federated AI represents a promising evolution in AI training methodologies, safeguarding sensitive information while promoting collaboration. As industries increasingly prioritize data privacy, solutions like Tiber will be invaluable in harnessing the full potential of AI technologies. Companies exploring AI collaborations should consider implementing federated learning initiatives not just to enhance model accuracy, but also to uphold the highest standards of data integrity.
Write A Comment